From DSaaS to System Insights
Alephnet’s data science as a service ecosystem empowers all companies to connect data to aspirational outcomes.
The data itself serves as a light in the dark revealing insights into how an organization actually operates day-to-day. This enables a shift from being reactive to proactive.
The complexity of data systems grows every day. Many companies have specific software for each function from Payroll, Accounting, HR, ERP, Billing, CRM, analytics, and industry-unique systems.
Data and technology stacks house growing amounts of fast-changing data, scattered across the organization, often with no standard structure and external sources of great value, not considered or captured at all.
Organizations are making some of their largest decisions based on limited data and are starving for useful insights.
A Digital Ecosystem with Transparent Data
Establishing a comprehensive 360º trustworthy view of data is foundational to companywide analytics.
The first step needed to achieve data-enabled decision making across an entire organization is to bring together current available industry systems and data under one roof. It is critical for every part of an organization to share necessary data to prevent data silos.
Gathering and unifying relevant data to deliver a single source of truth empowers the workforce with a consolidated view that saves time and elevates productivity.
One digital ecosystem hosting a digitized organization data version enables analytics, machine learning, and systems of insight.
Machine Learning
Machines can look at lots of high dimensions of data and determine patterns. If the machine can learn this pattern, it can discover anomalies, spot trends, and generate predictions based on learning. Once patterns are learned predictions can be made that humans can’t even come close to.
This is machine learning and part of the world of AI in which data scientists extract insights from data to solve complex challenges. The right data enables all manner of actionable insights across all business activities for quantifiable top and bottom-line impact.
Data scientists in multidisciplinary teams collaborate with stakeholders across the firm to;
- Design and test new ways to generate data
- Sift and prepare existing data
- Conduct exploratory data analysis, Al- Machine learning concept model identification, pattern and anomaly detection, and predictive model creation
- Identify and validate use cases for decision making
- Develop and validate process or business models
- Develop integration strategies into the operational business
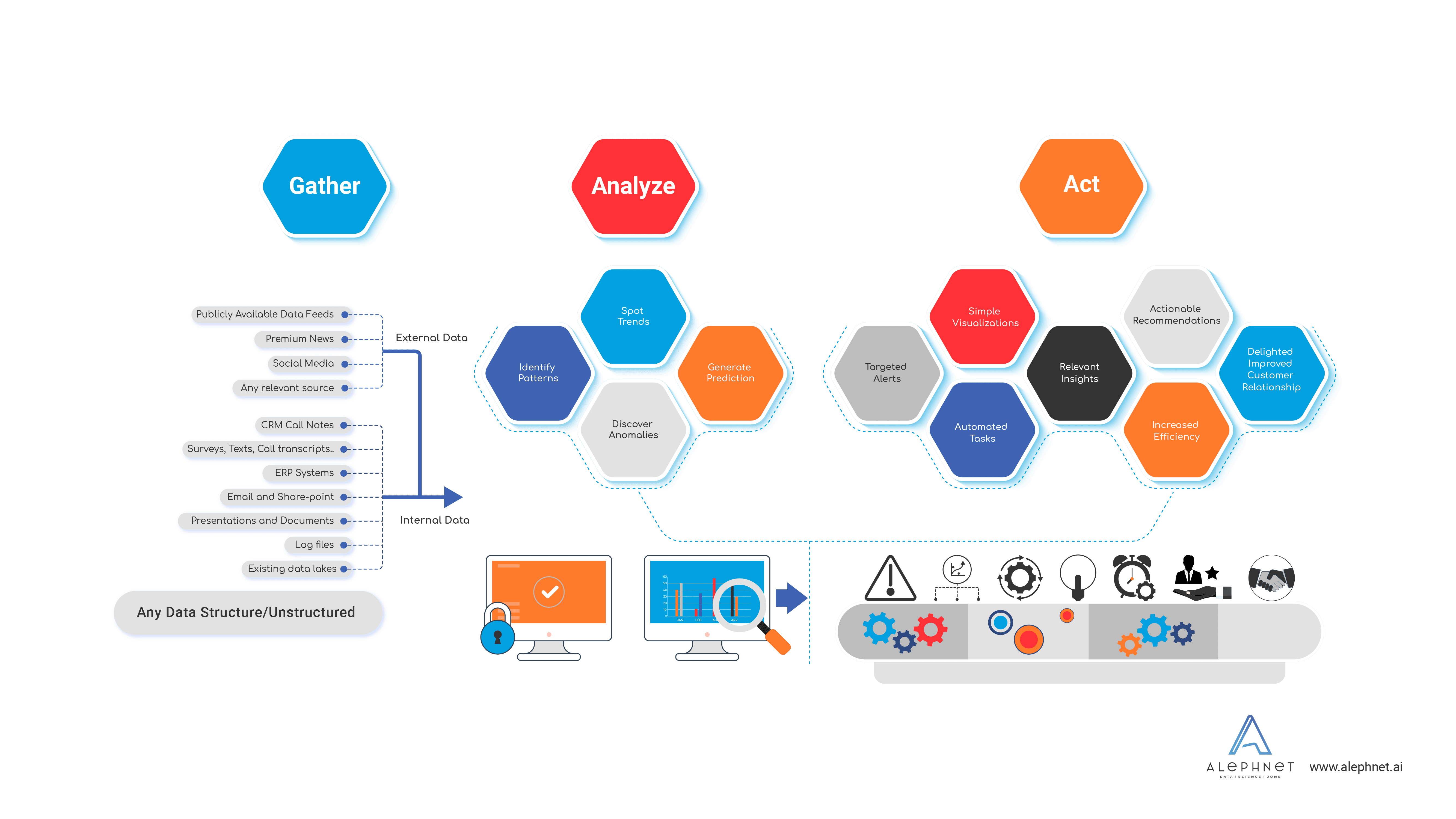
Organization data-enablement
Every company should be asking the question “How can data help us achieve our goals in all activities”?
To scale the extraction of value from data and connect it to valuable outcomes requires workforce integration. This change management and transformation requires organizations to focus on their people and build a culture that prioritizes data in decision-making, empowering the workforce to become data science citizens.
A consistent lean analytics approach provides an operating framework to support all stakeholders in this journey.
Workforce Adoption
Changing workplace habits isn’t as easy as flipping a switch. A workforce of data science citizens doesn’t just happen. Every organization faces challenges that a data strategy should help address.
Descriptive analytics enable organizations to answer what has happened in the past that produced current outcomes. Advances in descriptive analytics that make use of visual tools enable a shift towards self-service models where non-technical users can, in certain circumstances develop their own analyses.
Useful to an organization’s broader workforce, descriptive analytics still typically require time for a user to become proficient. This can lead to low employee adoption rates and or frequency of use.
Predictive and Prescriptive Analytics
Analytical approaches incorporating predictive models are progressively displacing descriptive approaches. Predictive analytics help answer the question of what might happen in the future. They help solve adoption challenges while also delivering deeper dimensions of insight than descriptive approaches.
Prescriptive analytics answer the question of what do we have to do to make it happen? Enterprise analytics utilizing AI incorporating both automation and contextual information results in the right insights being delivered to the right people at the right time automatically.
Automating Analytics
Automatically identifying relationships and patterns in data reduces the reliance on human expertise and judgment while keeping intentionality in the hands of a human actor. Augmenting intelligence, which is an alternative perspective of artificial intelligence that focuses on Al’s supportive role, emphasizes the fact that cognitive technology is intended to enhance human intelligence rather than replace it.
Incorporating role-based assumptions around decisions made on data and analytics alleviates the need for analysts to prepare in advance. Employees automatically receive relevant intelligence without having to invest more time which naturally aids adoption helping to solve a key challenge for most companies.
Automating data analytics changes the field of play, the makeup of team members that play, and the dynamics.
Digital Transformation
In the past, if you wanted to analyze supply chain, human resources, financial, or any business activity’s data you would first need to find the relevant data possibly scattered across siloed systems and direct the right analytics tool to it. This not only required extensive knowledge of what data was the right data, trustworthy, and pertinent to analysis but also where to locate it. Furthermore, analysts may have lacked the knowledge of the role and functional context necessary to connect to compelling outcomes.
Data science as a service empowers organizations to address the resourcing gaps and obstacles that have prevented the digital transformations necessary to compete in a digital economy. A system of insights brings data science to an entire organization.
Cognitive search is an example of contextual decision-making using artificial intelligence aligned to role-based assumptions to improve search queries and extract information from diverse data sources.
Cognitive search It goes beyond traditional search engine functionality by collating many data sources while enabling automated tagging and personalization. It elevates how an organization’s employees discover and access greater amounts of relevant information in the context of their work.
System of Insights
Each data-driven business decision-making problem is unique; comprising a combination of goals, desires, and constraints.
There are sets of common tasks that underlie a business problem. Recognizing familiar problems and solutions avoids wasting time, enabling greater attention on parts requiring expert involvement where human creativity and intelligence are paramount.
For this reason, we categorize insights across business activities such as; Risk, Services – incorporating operations, logistics, and procurement – Sales, Marketing, Talent, and Data discovery insights generally. Data science enables a companywide system of insights across all business activities.
Companies need to accelerate a move towards digitization and consider how to leverage new technologies and data science expertise to allow for a faster flow of data.
Broad companywide insights
In a digital economy, the traditional interrelated value chain activities are fluid and evolving at speed.
The ability to generate real-time insights draw the intersection of roles and function ever closer, blurring and redefining responsibilities and accountabilities across the entire value chain.
Most people are at least vaguely aware of the evolution of agile digital marketing;
“Using data and analytics to continuously source promising opportunities or solutions to problems in real-time, deploying tests quickly, evaluating results, and rapidly iterating.”
Insights from data help marketers understand how to attract new customers and delight existing clients. Data scientists help by classifying past and present data, detecting patterns, anomalies, and trends, and identifying relevant models to predict the future. Insights across interrelated value chain activities and functions of marketing, sales, and service help organizations identify demand, develop and create new services, products, and experiences. Revenue teams are primed with intelligence on market trends, shifting customer demand, pricing appetite, discovering what customers want now and what they will want in the future, and with predictive modeling even which customers are most likely to leave and why?
Revenue operations
(RevOps) is an example of the convergence of interrelated roles made possible by the speed and volume of data production, and the ability to ingest and drive insightful business intelligence.
Revenue operations are the strategic integration of revenue-related roles, sales, marketing, and service departments. The main goal of RevOps is to connect data from these departments to provide a better 360º customer view before, during, and after the sale. As digital customer experience evolves, the need for various departments to share information has grown. RevOps is the strategy to bring them all together to precisely manage and measure the return on investment (ROI). It takes responsibility for the software, systems, processes, and data for all the revenue-generating teams inside a company.
Valuable outcomes from previously unseen insights are waiting to be discovered across the entire value chain. Insights with collective relevance across interrelated functions just as with RebvOps; the supply chain, interrelated activities of procurement, internal and external logistics operations, and human resources, the entire companywide value chain.
Supply chain
Typically when planning, purchasing, and production departments work in silos, information gets delayed or goes un-communicated, slowing down the process and resulting in part or completely uninformed decision-making. Synchronizing data provides opportunities for transparency and collaboration. Shared data leads to faster response times and a more efficient process overall.
Departments can have more frequent and real-time access to information across all channels, allowing for better evaluations and informed decision-making. Such data synchronization reduces redundancies, improves communication, and offers a clearer insight into channels. It also gives companies the advantage of better predicting demand and identifying risks, making forecasting and risk assessments more accurate and helping to meet demand with efficient effective supply.
Regardless of business function from Sales, Marketing, Operations, Services, to Human Resources and Risk management a single source of trustworthy data, secure and governed empowers an organization to derive actionable business intelligence for a competitive edge.
Alephnet operates on the leading edge in this space, advancing the possibilities of cognitive networks to solve the most complex challenges. Reasoning approaches add to the knowledge that organizations have for their particular domain issues, accelerating their ability to analyze, learn about, and overcome them through a cycle of discovery. Valuable to all industries with applications across all business activities, departments, and locations companies can augment human intelligence enabling a workforce to do more
The use cases for Data Science, AI, and insights are endless
Designing systems of insight
A critical skill for a data scientist is the ability to decompose a data analytics problem into pieces that match a known task for which tools are available. The Data Science and AI landscape provide a deeper dive into the evolving, growing battle for resources.
Together let’s find your use cases and start to build your systems of insight.
Turn data into actionable insights
