What is Data Science?
Alephnet’s data science services turn your data into powerful outcomes.
Data science is a comprehensive term encapsulating the intricate, multi-step processes used to extract value from data.
Data science empowers us to meaningfully utilize vast amounts of information, gaining insights that enable us to do more.
Data scientists employ various forms of artificial intelligence to link data insights with valuable outcomes.
It’s a Digital World
With 4.388 bn internet users, 5.112 bn mobile users & 3.484 bn active social media users worldwide.
-Global Digital Reports 2020
In an era where information is abundant and widely circulated, the ability to capture, analyze, and respond to context-specific insights has become imperative. Data is now the driving force behind numerous global industries and innovations.
Leaders who incorporate data science and AI into their business and company culture are creating systems with actionable insights, giving them a competitive edge.
The Rise of Data Science and AI
Despite AI’s existence since the 1950s, its practical application has only recently come to light. The last four years have seen a significant upswing in AI investments.
The emergence and growth of data science and AI are largely due to three key factors:
- Unprecedented access to extensive data from e-commerce, businesses, government bodies, academia, wearables, and social media.
- The refinement of machine learning (ML) algorithms, driven by the sheer volume of accessible data.
- The surge in computational power and the proliferation of cloud services, simplifying the execution of complex machine learning algorithms.
Data Science Advantaged
From tech giants like Google, Amazon, and Facebook to venture-capital-fueled startups like Uber on their journey to unicorn status, data science-based, data-driven business models are the engines of their performance.
They harness data science to refine every business decision. They see insights, not just data, as a business commodity, creating a holistic data view: real-time insights enabling immediate, context-specific actions across all business operations for superior results. They are dynamically poised to captivate customers, bolster relationships, and secure a competitive advantage.
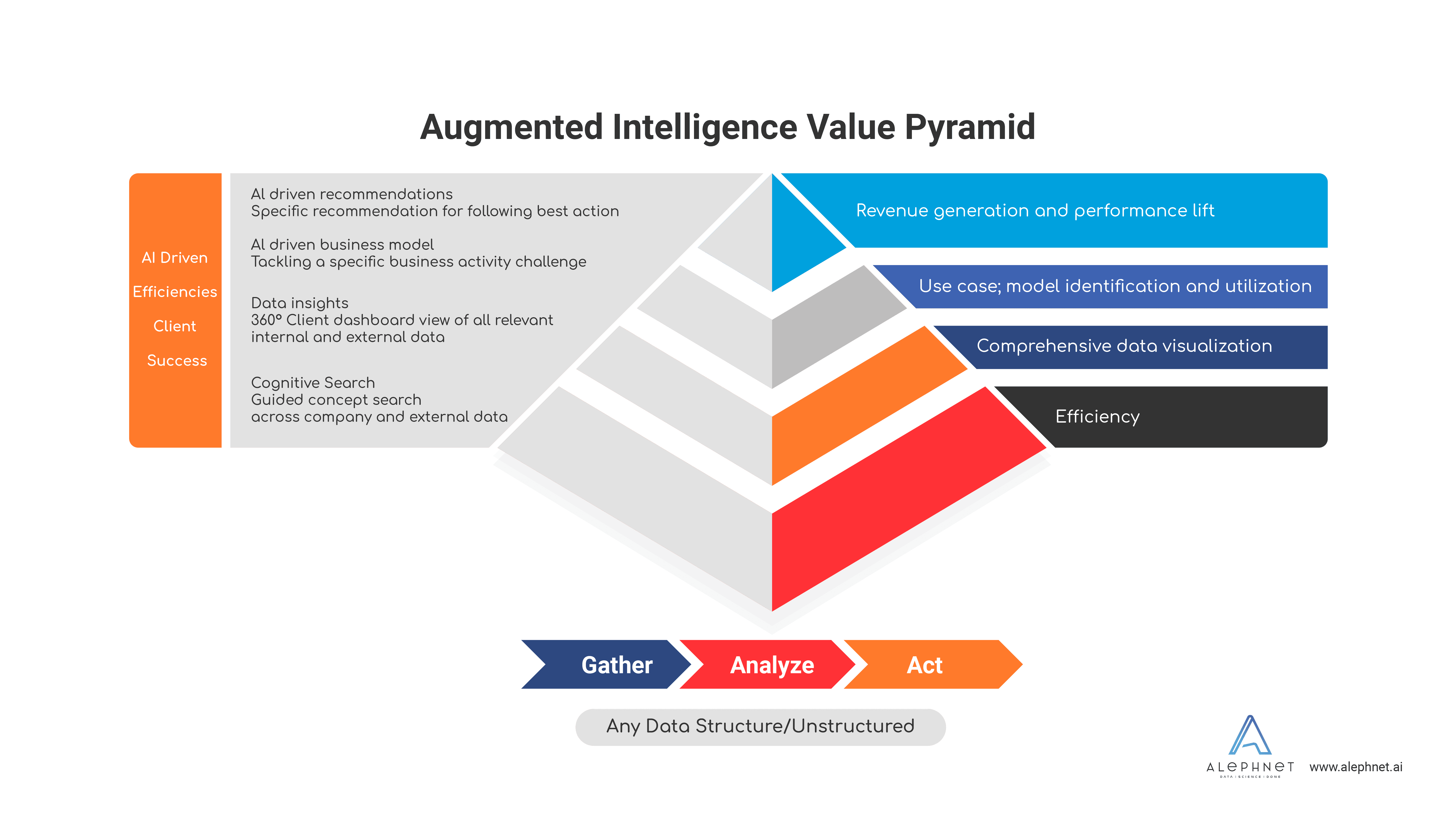
Data Science Use Cases
A singular, reliable data source equips an organization with the analytical prowess to extract actionable business intelligence across all operations.
Data analytics pave the way for continuous opportunity sourcing, real-time problem-solving, test case deployment, result evaluation, and iteration.
With Data Science and AI, the Possibilities are Endless
The capacity to generate real-time insights is causing roles and functions to increasingly overlap, leading to a blurring and redefinition of responsibilities and jurisdictions.
Traditional activities in the value chain are evolving at a rapid pace, as is the competitive landscape, where competition is shifting from specific industries to comprehensive ecosystems.
This is evident in the transition from cars to mobility solutions. Companies like Spotify are a prime example of how user services have entered the mainstream. Consumers are now paying for temporary access to things they used to buy outright, all with hyper-personalization.
From banking to fintech platforms, from production lines to smart factories – traditional boundaries are collapsing almost everywhere. Competitors are emerging from new directions, pursuing different objectives than familiar rivals.
Data Science and the use of AI is Industry Agnostic
Retail outlets are harnessing real-time insights to update and optimize their pricing while leveraging machine learning with weather data to forecast product demand. Combined with automated inventory management and competitor pricing, businesses ensure the right product is available at the right time and price to enhance sales.
Corporations are automating procurement management to optimize supplier expenditure.
Banks are streamlining customer lending through automation. Unconventional transaction data, captured by predictive AI algorithms, facilitates superior credit quality evaluation. This efficiency reduces loan defaults and boosts credit portfolio growth and performance.
Advanced intelligence in healthcare is already a lifesaver, enhancing the accuracy of heart failure detection by emergency call handlers. Natural language processing and other AI forms translate verbal and non-verbal cues like tone and breathing pattern. With data from millions of past emergency calls, AI models can identify signs of cardiac arrest, alert and provide recommendations to the handler during the call. This has led to a more than 20% increase in accurate and swift heart attack diagnosis, saving numerous lives.
A Data Science Framework
In a world marked by ecosystem disruptions, businesses need an innovative strategic approach. An insights ecosystem equips a company to foster innovation, attain business agility, and thrive in the digital economy.
Insight-Driven Companies are more Agile and Resilient
Average growth rates of insights enabled companies
Public 27% CAGR
Private 40% CAGR
Average growth rates without insights
GDP +3.6% CAGR
* Sources: Forrester, Morningstar, PitchBook, The Economist Intelligence Unit; See also: “The Insights-Driven Business” Forrester report
Insight-driven companies are
23 X more likely to acquire customers
19 X more likely to be profitable
2.6 X more likely to exceed competitor’s ROI
* Sources: IDC, Gartner Forrester 2019 / 2020
44% of larger organizations fear they’ll lose out to startups if they’re too slow to evaluate and deploy Al in their organization
– Microsoft Digital Research
It is expected that the global artificial intelligence market will grow at a compound annual growth rate of 42.2% from 2020 to 2027 to reach USD 733.6 billion by the end of 2027
– Insights from Grandview Research
Data Science Process
Transforming data into actionable insights can lead to enhanced efficiency, cost savings, increased productivity, employee engagement, revenue growth, and overall company value. This can be achieved through a simple three-step method:
Gather, Analyze, and Act.
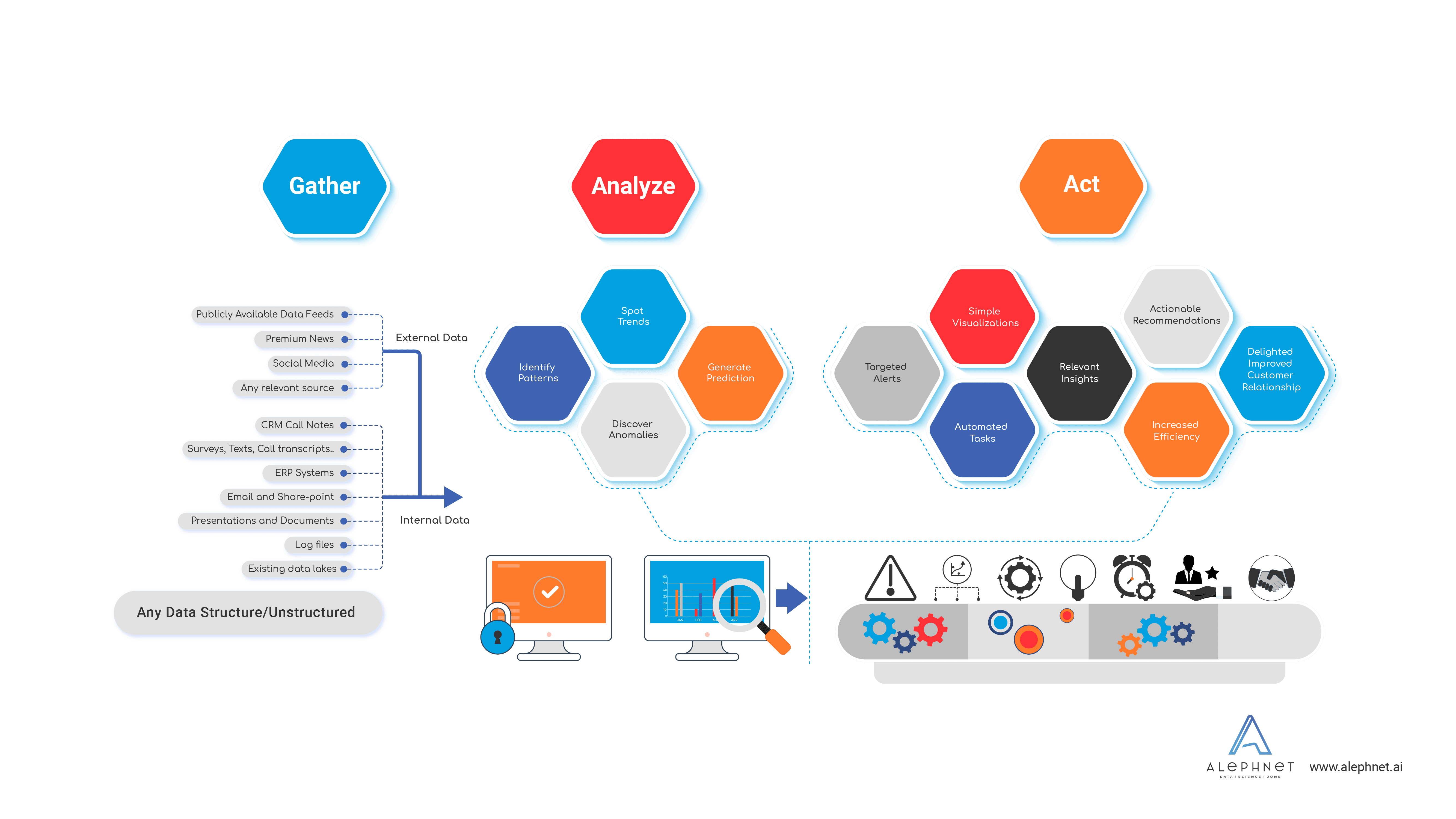
Smart outcomes
- Automation: Enhanced efficiency, lower costs, increased productivity
- Anomaly and Trend Detection: Early identification of threats and risks
- Auto Discovery: Gaining insights to empower the workforce
- Catalyst Detection: Benchmarking, R&D, advanced deep learning
- Enrichment Pipeline: Fostering new opportunities, services, and products
- Smart Recommendations: Advanced intelligence
- Insight System: A comprehensive approach to data analysis and interpretation
Key Barriers to Entry and Challenges in Data Science

Data Science - Barriers to Entry And Digital Challenges
Barriers to entry and digital challenges
To compete in the digital economy, organizations must overcome a range of challenges
- The need for awareness and a cultural mindset that embraces data utilization
- A willingness to invest in resources and workforce adoption
- Challenges related to data access, quality, activation, and analytics application
- The impact of growing regulations
- The availability of frontend and backend resources
Other trends in Data Science and AI
Organizational Culture Data Maturity
How can companies align their decision-making processes more effectively with data? What specific commitments do successful companies make in order to invest in the necessary resources for achieving their goals? These commitments include investing in talent, processes, and a wide range of technologies that enable the widespread implementation and use of data and analytics.
According to research conducted by IDC, 83% of CEOs express a desire to make their companies more data-driven, and data-driven companies with a mature data culture achieve better results by improving their business metrics and achieving better outcomes.
The benefits of aligning decision-making processes with data include:
- Stronger differentiation from competitors
- Faster time to market
- Higher profits
- Increased employee satisfaction
Harness the Power of Data: Boost Business Value Through a Data-Driven Culture
In today’s digital economy, it’s essential for leaders to transform their business with a focus on developing agility and resilience.
Today’s most successful companies are embracing a data-driven culture. They strive to extract maximum value from their data and foster a culture of data exploration and curiosity. They recognize the value of data by empowering their entire workforce at all levels to make better data-based decisions. They make data literacy a priority and work to break down organizational silos to promote collaboration and develop enterprise-wide systems for insights.
Companies that highly value a data-driven culture empower data-literate talents to leverage data science to seize opportunities, drive growth and innovation, and set themselves apart from the competition.
Data Access, Quality, and Activation Challenges
Companies Face Growing Complexity of Systems
Many companies have specific software for each function – from Payroll, Accounting, HR, ERP, Billing, CRM, analytics to industry-specific systems. Data and technology stacks house growing amounts of fast-changing data, scattered across the organization, often without a standard structure and external sources of great value, not considered or captured at all.
Up to 95% of all data is unstructured – social media content, call transcripts, video, audio, and much more.
Unstructured data is often where the most insight is mined, but it’s also largely unused. As a result, most organizations use a mere 1% of available data to help improve decisions. Source: Hopkins et al. Forrester.
Impact
- Cumbersome access consumes a lot of time.
- Up to 30% of an employee’s workday can be spent searching and gathering data – 2 ½ hours per day
- Missing information hampers business application
- Opportunity loss due to inability to find relevant data
- Productivity loss
- prone to errors
- data duplication
- shifts concern from missing data to bad data.
Companies attempt to empower their workforce, make them citizen data scientists, only to fail partly because the approach has been technology-focused and the skillsets necessary to manage a data product lifecycle are broad and uncommon.
Growing Regulations
Data science and the use of AI bring unlimited potential, but with great potential comes significant risks.
Using data science and AI to support business-critical decisions makes it vital to understand what AI is doing and why. Is it providing valuable contextual insight or making accurate, bias-aware decisions? Does it violate privacy regulations? Can you govern and monitor the models to ensure compliance with progressive regulations?
AI development by someone without the expertise and proper training, or operating without appropriate controls can create something dangerous; not being able to explain the model’s results, problems may become evident after system implementation, leaving companies with severe liability. Even the most sophisticated AI systems of highly qualified engineers can cause explainability reinforcement risks and unintended consequences.
AI should be designed, tested, and maintained by people with relevant expertise.
When organizations consider how to implement and maximize the use of data science and AI, they must be aware of the demand and difficulty of attracting and retaining data scientists. This impacts not only the targeted nature and quality of outcomes but also the ability to manage and mitigate growing risks.
The ‘Data-Science as a Service’ platform elegantly empowers all companies to solve these growing, critical pain points and drive a return on data.
Access to Frontend and Backend Resources
Data science and the use of AI is not a choice anymore, it’s necessary for long-term success. To succeed, companies need to utilize technology, knowledge, computational power, access to data, and the expertise to execute.
AI requires data, and data without context is really just noise. Despite much progress, it’s still difficult to use data and analytics to understand and predict many of the important phenomena in organizations. Predictive models require a substantial amount of past data and knowledge to create and use, so without the necessary resources, they can be limited to how, when, or if they can be deployed.
There are many backend tools and solutions designed to extract value from data. The backend is the part that deals with hardware, efficient computing, and data storage infrastructure, or what is often referred to as data engineering.
The frontend data scientist(s) landscape is more challenging.
The Data Science & AI Landscape – Tech & Data Scientist Spectrum provides a deeper dive into the evolving landscape and battle for resources.
Solution
Data studio as a service provides the framework and roadmap for businesses to accelerate the adoption and benefits of data science.
The service includes three modular solutions tailored to the specific needs of each customer, providing progressive value and benefit in each part.
They are part of the broader DSaaS ecosystem, which allows all businesses access to demand and subscription-based data science front and backend resources, thereby creating a level playing field.